Patents have long been a cornerstone of innovation and economic growth, serving as powerful tools for protecting intellectual property. However, with the growing number of patent applications worldwide, the need for efficient and effective patent prioritization strategies has become more critical than ever. In this article, we delve deep into the world of AI-powered patent prioritization strategies, exploring how artificial intelligence is transforming the way inventors and businesses navigate the patent landscape.
Understanding Patent Prioritization
Patent prioritization is the process by which inventors and businesses determine which inventions to prioritize for patent protection. It involves evaluating the novelty, utility, and potential market value of an invention to decide whether to pursue a patent. The goal is to focus resources on protecting the most valuable intellectual property.
Why is patent prioritization important?
Benefits for Inventors and Businesses
Patenting can be an expensive and time-consuming process. Prioritization ensures that resources are allocated wisely to protect innovations with the highest potential for commercial success.
The Competitive Advantage of Timely Patents
In today’s fast-paced business environment, securing patents quickly can provide a competitive edge. Patent prioritization ensures that promising inventions are not delayed in the application process.
The Traditional Patent Prioritization Process
In the past, patent prioritization was often a manual and subjective process. Inventors and patent attorneys would review inventions based on their expertise and intuition, leading to potential biases and errors.
Limitations and Challenges
- Subjectivity and Bias
Human evaluators might have personal preferences or biases, impacting which inventions get prioritized. This subjectivity can lead to missed opportunities or unnecessary patenting costs.
- Time-Consuming Nature
Manual evaluation is a slow and labor-intensive process, causing delays in patent filings. In fast-moving industries, this can be detrimental to a company’s competitiveness.
The Rise of AI in Patent Prioritization
Artificial Intelligence (AI) encompasses technologies that enable computers to perform tasks that typically require human intelligence, such as learning, reasoning, and problem-solving. In patent prioritization, AI algorithms can analyze vast amounts of data quickly and make objective decisions based on predefined criteria.
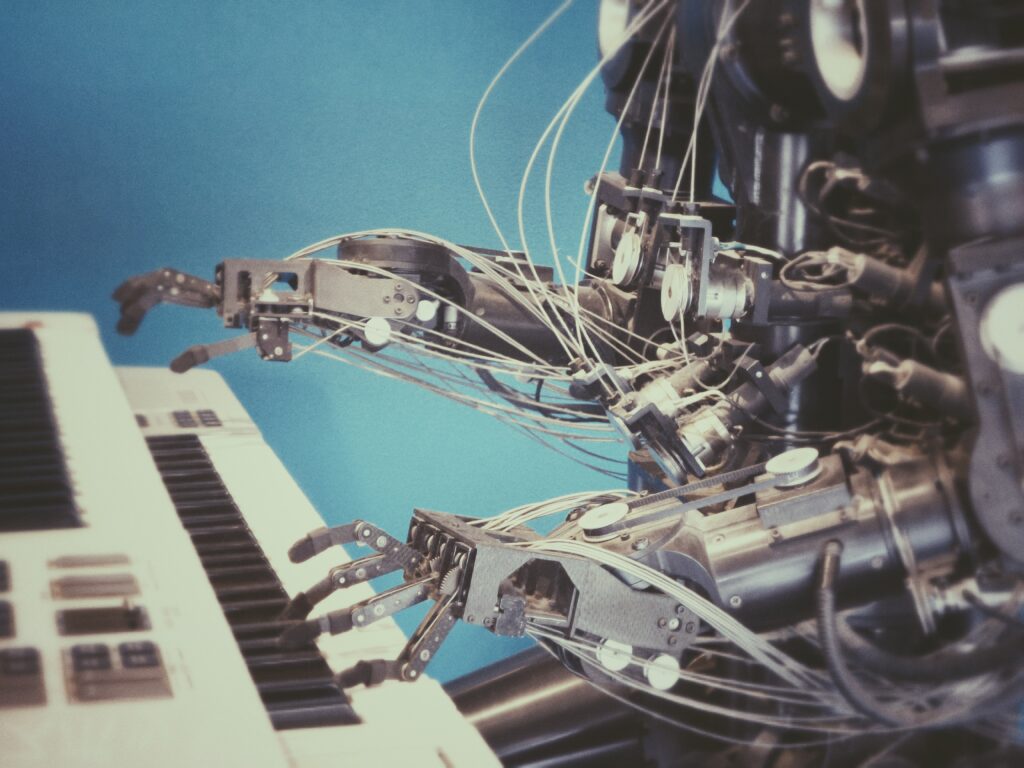
Advantages of AI-Powered Patent Prioritization
Speed and Efficiency
AI algorithms can process and analyze large datasets in seconds, dramatically reducing the time required for patent prioritization.
Objectivity and Reduced Bias
AI algorithms make decisions based on data, eliminating the potential for personal bias in the prioritization process.
Handling Large Volumes of Data
In today’s digital age, patent databases and scientific literature are constantly growing. AI can handle the vast volume of information available, ensuring no valuable data goes unnoticed.
AI Tools for Patent Prioritization
Artificial Intelligence (AI) has revolutionized the field of patent prioritization by introducing powerful tools that can handle the vast volume of data involved in the patent application process. These tools rely on advanced machine learning algorithms and data sources to provide patent professionals with valuable insights for effective decision-making.
Machine Learning Algorithms
One of the key components of AI tools for patent prioritization is the utilization of machine learning algorithms. These algorithms can be tailored to specific tasks within the patent prioritization process, such as Natural Language Processing (NLP) and image recognition.
Natural Language Processing (NLP)
NLP is a game-changer in the field of patent prioritization. It allows AI algorithms to process and analyze the textual content of patent applications and related documents. This is especially useful for extracting essential information about inventions, their novelty, and potential market impact.
NLP can identify and understand the technical language used in patents, making it possible to uncover critical insights that might not be apparent through manual review. It can also identify relationships between patents, technologies, and inventors, enabling patent professionals to make informed decisions.
Image Recognition
In fields like design patents and medical imaging, visual elements play a significant role in invention disclosure. AI-powered image recognition algorithms can be employed to analyze and categorize visual content within patents. These algorithms can identify design patterns, shapes, and structures, enabling efficient sorting and classification of patents based on visual data. This capability is invaluable when prioritizing patents in industries where the visual aspect of the invention is a primary consideration.
Data Sources for AI-Powered Prioritization
AI tools draw their power from the data they can access and analyze. In the realm of patent prioritization, this data is sourced from various repositories and databases, including:
Patent Databases
AI algorithms can crawl patent databases such as the United States Patent and Trademark Office (USPTO), the European Patent Office (EPO), and other national or international patent offices. These databases contain a treasure trove of information, including existing patents, applications, and historical patent data. AI can efficiently extract, categorize, and analyze this data to identify trends, assess the novelty of inventions, and make informed recommendations for patent prioritization.
Scientific Literature
In addition to patent databases, AI tools can access a vast array of scientific literature, including academic journals, conference papers, research articles, and technical reports. This literature often contains valuable insights into emerging technologies, scientific developments, and potential innovations. AI-powered tools can comb through this extensive collection of documents to identify connections between academic research and patent activity, providing a comprehensive understanding of the intellectual property landscape.
Market Trends and Analysis
AI can also tap into market trends and analysis data. This includes data related to industry trends, consumer preferences, market demand, and competitive landscapes. By integrating market data with patent-related information, AI tools can help inventors and businesses assess the commercial potential of their inventions. This critical insight enables patent professionals to prioritize inventions that align with market trends and have a higher probability of economic success.
Strategies for Implementing AI-Powered Patent Prioritization
Implementing AI in patent prioritization requires a well-planned approach, involving various stages from data collection to integration into existing patent workflows. Here’s a detailed breakdown of these strategies:
Data Collection and Preprocessing
- Collecting Relevant Data: The foundation of AI-powered patent prioritization is data. Begin by collecting data from a wide array of sources, including patent databases, scientific literature, and market trend reports. Ensure the data is comprehensive and up-to-date to provide accurate insights.
- Data Preprocessing: Raw data can be messy and inconsistent. Preprocessing involves cleaning and structuring the data. This may include removing duplicates, standardizing formats, and enriching data with relevant metadata, such as inventor details, application dates, and technology categories.
- Data Quality Assurance: Quality control is crucial. Implement procedures to validate data for accuracy and completeness. Data that is riddled with errors can lead to incorrect prioritization decisions.
Choosing the Right AI Tools and Platforms
- Selecting AI Algorithms: Choose machine learning algorithms that best suit your patent prioritization needs. For example, if dealing with textual patent data, Natural Language Processing (NLP) algorithms are essential. Image recognition algorithms are critical for visual patent content.
- Customization: Tailor the AI algorithms to specific requirements. Train the algorithms on a dataset of known, well-prioritized patents to align them with your organization’s priorities and criteria.
- Scalability: Ensure that the chosen AI tools and platforms can handle the scale of data and complexity of your patent portfolio. Scalability is crucial to accommodate both current and future needs.
Building a Skilled AI Team
- AI Experts: Employ professionals with expertise in AI, machine learning, and data science. Their knowledge is vital for customizing and fine-tuning the algorithms.
- Legal and IP Expertise: Integrate legal and intellectual property experts into the team. They should understand the intricacies of patent law, patent application processes, and the legal implications of AI decisions in patent prioritization.
- Data Analysts: Data analysts are essential for data preprocessing, ensuring data quality, and extracting meaningful insights from the AI-driven analysis.
Integration with Existing Patent Workflows
- Seamless Integration: The AI-powered patent prioritization system should be seamlessly integrated into your existing patent workflows. It should be user-friendly, allowing patent professionals to interact with it easily.
- User Training: Provide training for your patent professionals on how to use the AI tools effectively. They should understand how the system works and how to interpret the insights it provides.
- Continuous Monitoring and Feedback: Establish a feedback loop to continually monitor the AI system’s performance. Patent professionals’ feedback is valuable in fine-tuning the system and ensuring its ongoing relevance.
- Compliance with Patent Laws: Ensure that the AI system complies with patent laws, regulations, and guidelines. It should assist in identifying patentable inventions while avoiding potential infringement risks. Implement robust security measures to protect sensitive patent-related data. Data breaches can have serious legal and financial consequences.
- Adaptation to Changing Needs: The patent landscape is dynamic. Your AI-powered patent prioritization system should be adaptable to changes in your organization’s patent strategy, market conditions, and technological developments.
Challenges and Ethical Considerations
Challenges in AI-Powered Patent Prioritization
Data Quality and Bias:
AI systems heavily rely on data, and if the data used is biased or of poor quality, it can lead to skewed results. Biased data can reinforce existing biases in patent prioritization, potentially favoring certain inventors, technologies, or regions. It is crucial to identify and mitigate bias in the data and algorithms to ensure fair and objective prioritization.
Data Privacy:
The use of AI in patent prioritization involves processing and analyzing vast amounts of data, including sensitive information. Protecting the privacy of inventors and other stakeholders is a significant concern. Striking a balance between data access for analysis and maintaining privacy is a complex challenge.
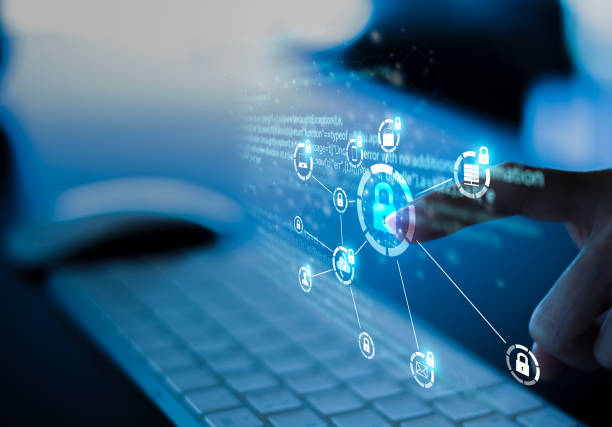
Regulatory Compliance:
Adhering to patent laws and regulations is paramount. AI systems must not only assist in identifying patentable inventions but also ensure that patent applications are in compliance with local and international patent laws. Staying abreast of evolving patent regulations presents an ongoing challenge.
Explainability and Transparency:
AI algorithms, especially deep learning models, can be complex and challenging to understand. The lack of transparency in AI decision-making raises concerns, as stakeholders may have difficulty comprehending why a particular patent was prioritized over another. Achieving transparency and explainability in AI models is an ongoing challenge.
Integration and Training:
Integrating AI systems into existing workflows and training patent professionals to use them effectively can be met with resistance and difficulties. Change management is often an overlooked challenge in adopting AI solutions for patent prioritization.
Ethical Considerations in AI-Powered Patent Prioritization
- Bias and Fairness: Ensuring that AI systems do not perpetuate or amplify existing biases is a fundamental ethical concern. Bias in prioritization can result in unfair advantages or disadvantages for certain inventors or technologies. Continuous efforts are required to develop and fine-tune AI models to reduce bias and ensure fairness.
- Privacy and Data Protection: Respecting the privacy of inventors and patent applicants is essential. Collecting and processing large volumes of data can raise privacy concerns. It is ethically imperative to establish robust data protection measures and obtain informed consent when necessary.
- Accountability and Responsibility: AI systems may make decisions autonomously, but ultimately, humans are responsible for their deployment and outcomes. Determining who is accountable for AI-driven patent prioritization decisions and potential errors is an ethical consideration that needs to be addressed.
- Transparency and Explainability: Ethical prioritization requires transparency and explainability. Stakeholders should be able to understand and question the decisions made by AI systems. Ethical AI systems should provide clear justifications for their recommendations.
- Accessibility and Inclusivity: AI-powered patent prioritization should be accessible to all inventors and organizations, regardless of their size or resources. Ethical considerations include ensuring that AI systems do not create disparities in access to patent protection or prioritize inventions from larger corporations disproportionately.
- Ethical Patent Enforcement: While patent prioritization aims to identify valuable innovations, it’s essential that ethical considerations extend to the ethical enforcement of patents. Ethical patent holders should use their intellectual property rights responsibly, avoiding frivolous litigation and fostering innovation.
Addressing these challenges and ethical considerations in AI-powered patent prioritization is essential to ensure that the technology is used responsibly and to the benefit of inventors, organizations, and society as a whole. Collaboration between AI experts, legal professionals, and stakeholders is crucial in creating guidelines and best practices that align with ethical standards and the evolving field of intellectual property.
Leave a Reply