Innovation is the cornerstone of progress, and the protection of intellectual property plays a pivotal role in fostering this innovation. Patents are legal documents that grant inventors exclusive rights to their creations for a specific period, in exchange for sharing their knowledge with the world. Understanding the patent landscape, with its ever-growing complexity, is no small feat.
It involves extensive research, analysis, and a keen understanding of legal intricacies. Traditional methods of patent analysis can be time-consuming and costly. Fortunately, the advent of artificial intelligence (AI) has revolutionized the way we analyze patents. We’ll explore the multifaceted landscape of AI’s role in automated patent analysis reports, delving deep into its evolution, advantages, challenges, and real-world applications.
Understanding Patent Analysis
Patent analysis is a comprehensive process involving the evaluation of patents’ legal, technical, and commercial aspects. It provides vital insights into the innovation landscape, helping inventors, businesses, and policymakers make informed decisions. This analysis goes beyond merely reading patent documents; it includes evaluating the patent’s legal strength, assessing its commercial potential, and mapping it to existing technology trends.
Patent analysis plays a pivotal role in various industries, including technology, pharmaceuticals, and manufacturing, to name a few. It helps businesses understand their competitive landscape, identify opportunities for innovation, and make strategic decisions. This process is also invaluable for legal professionals, ensuring that patents comply with legal requirements and are free from infringement issues.
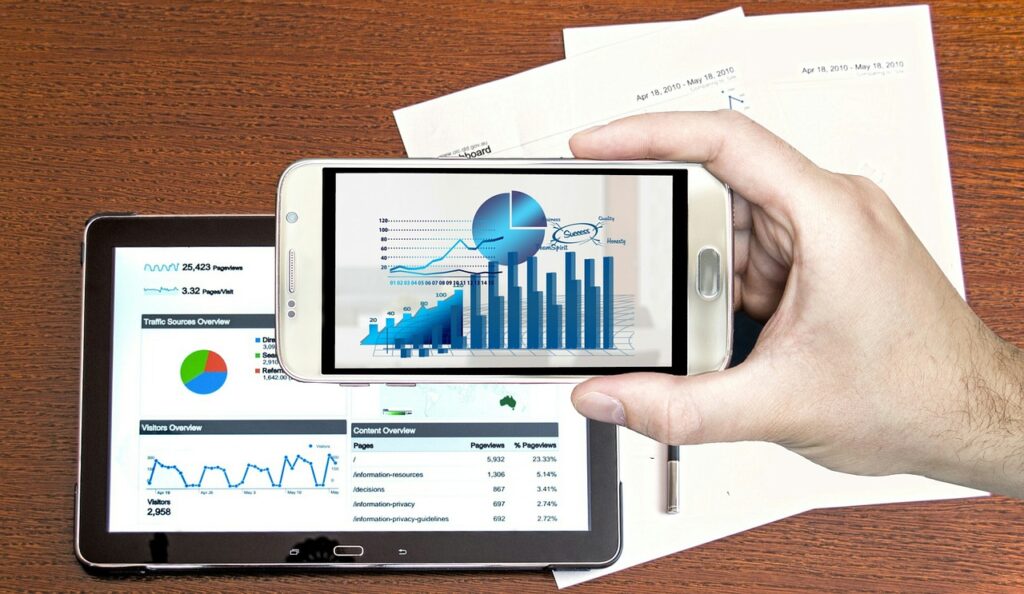
The Importance of Patent Analysis in Various Industries
The significance of patent analysis is not limited to a single industry. It extends its influence to various sectors where innovation is a driving force. Here are some examples:
1. Pharmaceutical Industry
In the pharmaceutical industry, patent analysis is crucial for understanding the competitive landscape and identifying opportunities for new drug development. It aids in avoiding patent infringement issues and contributes to the development of generic versions of drugs when patents expire.
2. Technology and Software Sectors
In the technology and software sectors, patents are vital to protect intellectual property. Patent analysis helps companies understand the strengths and weaknesses of their patents, enabling them to make strategic decisions regarding litigation, licensing, and innovation.
3. Automotive and Manufacturing Sectors
In the automotive and manufacturing sectors, patent analysis is essential for keeping up with technological advancements. It helps companies identify new materials, processes, and designs that can give them a competitive edge.
Traditional Patent Analysis Methods and Their Limitations
Before AI’s foray into patent analysis, traditional methods involved manual review and analysis of patent documents. Experts with knowledge of both the technical and legal aspects of patents were essential for this process. These experts would spend countless hours reading through patent documents, assessing their claims, and providing insights.
Limitations of Traditional Methods
While these methods have served their purpose, they come with several limitations:
- Time-Consuming: Manual review is labor-intensive and time-consuming. It often takes weeks or even months to analyze a single patent portfolio comprehensively.
- Costly: Hiring experts to perform manual patent analysis can be expensive, making it a resource-intensive process.
- Subjectivity: Manual analysis is prone to subjectivity and human errors. Experts’ interpretations may vary, leading to inconsistencies in the analysis.
- Scalability: Traditional methods are not easily scalable. As the number of patents continues to grow, it becomes increasingly challenging to keep up with the pace.
- Inefficiency: The vast amount of data contained in patent documents makes it nearly impossible for humans to perform in-depth analysis efficiently.
The limitations of traditional methods paved the way for the integration of AI in patent analysis. AI systems, with their capacity to process vast amounts of data, perform complex tasks, and adapt to evolving trends, have revolutionized the patent analysis landscape.
The Role of AI in Patent Analysis
Artificial intelligence has come a long way since its inception. The last few decades have witnessed remarkable advancements in AI technology. In the context of intellectual property, AI has proven to be a game-changer. Its applications extend beyond patent analysis to other areas like trademark searches, copyright analysis, and even predicting IP trends.
AI’s impact on intellectual property stems from its ability to handle massive amounts of data, identify patterns, and make data-driven decisions. This makes it an ideal candidate for automating and enhancing patent analysis.
Advantages of Using AI in Patent Analysis
The integration of AI in patent analysis offers numerous advantages:
1. Speed and Efficiency
AI can process vast patent datasets in a fraction of the time it would take a human. This not only accelerates the analysis process but also allows for timely decision-making.
2. Scalability
AI systems can scale effortlessly, making it possible to analyze a large number of patents simultaneously. This scalability is especially valuable in an era where the volume of patent applications is steadily increasing.
3. Consistency and Objectivity
Unlike human analysts, AI systems are consistent and objective. They interpret data based on predefined algorithms, reducing the risk of subjective bias.
4. Pattern Recognition
AI excels in pattern recognition, allowing it to identify trends, emerging technologies, and potential infringements more effectively than traditional methods.
5. Cost Savings
By automating a significant portion of the patent analysis process, businesses and inventors can significantly reduce their expenses, making IP management more cost-effective.
Types of AI Technologies Employed in Patent Analysis
AI’s prowess in patent analysis is driven by various technologies. Let’s explore the primary AI technologies employed in this field.
1. Natural Language Processing (NLP)
NLP allows AI systems to understand and analyze human language. In patent analysis, it’s crucial for interpreting the complex language used in patent documents. NLP systems can extract essential information, including patent claims and descriptions, and convert it into structured data for further analysis.
2. Machine Learning (ML)
ML algorithms enable AI systems to learn from historical data and make predictions or classifications based on patterns. In patent analysis, ML can be used to categorize patents, predict future trends, and even identify potential patent infringements.
3. Deep Learning
Deep learning is a subset of machine learning that involves neural networks with multiple layers. It’s especially valuable for tasks like image recognition in patents, where it can identify specific diagrams or figures related to the invention.
4. Computer Vision
Computer vision is another AI technology used in patent analysis. It enables AI systems to analyze visual data from patent documents, such as drawings and diagrams. This technology is particularly useful for understanding the technical aspects of inventions.
Key Challenges in AI-Powered Patent Analysis
While AI has made remarkable strides in patent analysis, it’s not without its challenges. Let’s explore some of the key hurdles in implementing AI in this domain.
Data Quality and Quantity
AI’s effectiveness in patent analysis relies heavily on the quality and quantity of data available. Inaccurate or incomplete data can lead to flawed analysis and erroneous conclusions. Additionally, the sheer volume of patent data can be overwhelming, making it challenging to curate and maintain high-quality datasets.
Legal and Ethical Considerations
The legal and ethical aspects of patent analysis present complex challenges. AI systems must respect intellectual property rights and adhere to privacy laws. Furthermore, determining the legality of AI-generated patents raises questions about the role of AI as an inventor and the associated rights and responsibilities.
Interpretation of Complex Patents
Many patents involve intricate technical language, which can be challenging for AI systems to interpret accurately. Understanding the nuances and subtleties of these patents requires advanced natural language processing and domain-specific knowledge.
Overcoming Language Barriers in International Patents
International patents often come in various languages, presenting a language barrier for AI systems. Ensuring that AI can accurately analyze patents in multiple languages is a significant challenge for the global patent landscape.
While these challenges exist, ongoing research and development are aimed at addressing them. The potential benefits of AI in patent analysis make it a field ripe for innovation and solutions to these challenges.
Tools and Platforms for AI-Powered Patent Analysis
The adoption of AI in patent analysis has given rise to a plethora of tools and platforms designed to streamline the process. Let’s explore some of the prominent tools and platforms that are leading the charge in this space.
Overview of Popular AI-Based Patent Analysis Tools
1. PatSnap
PatSnap is a comprehensive patent analysis platform that combines AI and machine learning technologies. It provides features for patent search, competitive analysis, technology mapping, and trend identification. PatSnap’s AI capabilities help users uncover hidden insights in patent data.
2. Derwent Innovation
Derwent Innovation, powered by Clarivate Analytics, offers AI-driven tools for patent research and analysis. It aids in identifying patent opportunities, tracking competitors, and assessing the patent landscape. Derwent’s AI algorithms help users make data-driven decisions.
3. IBM Watson for Patents
IBM Watson for Patents is an AI-powered tool that leverages Watson’s NLP capabilities to interpret patent language. It assists users in conducting patent searches and analyzing patent portfolios. IBM Watson’s AI-driven insights can reveal valuable information for inventors and businesses.
4. Google Patents
Google Patents is a free platform that utilizes AI and machine learning for patent search and analysis. It offers powerful search capabilities, making it easier to find relevant patents. The AI algorithms help users narrow down their search and assess patent quality.
Features and Capabilities of Leading Platforms
AI-Powered Search
AI-driven patent analysis platforms provide advanced search capabilities. Users can conduct highly targeted searches to identify relevant patents quickly. The AI algorithms understand the user’s query and return accurate results.
Competitive Analysis
These platforms offer tools for competitive analysis, allowing businesses to gain insights into their competitors’ patent portfolios. AI can highlight areas of potential collaboration, as well as uncover potential infringement risks.
Technology Mapping
AI can create technology maps, which illustrate the relationships between various patents and technologies. This visualization helps users identify emerging trends and technological clusters.
Trend Identification
AI can identify trends within patent datasets. It can analyze the evolution of technology over time and predict future innovations. This insight is invaluable for businesses looking to stay ahead of the curve.
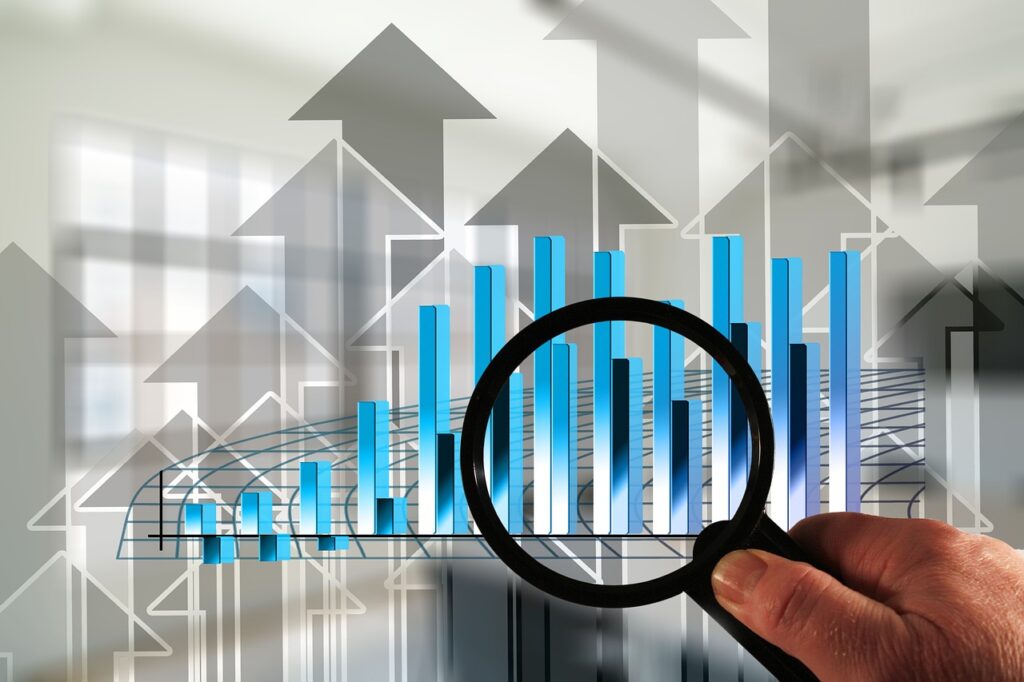
Predictive Analytics
Some platforms use AI for predictive analytics. By analyzing historical patent data, these tools can make predictions about future trends, patent values, and potential areas for innovation.
A Comparison of Open-Source and Commercial Solutions
The market for AI-powered patent analysis offers both open-source and commercial solutions. Here’s a brief comparison of the two:
Open-Source Solutions
- Cost: Open-source solutions are often free or have lower initial costs.
- Customization: Users have more flexibility to customize open-source tools to their specific needs.
- Community Support: Open-source tools may benefit from a community of users who contribute to their development.
Commercial Solutions
- Comprehensive Features: Commercial solutions tend to offer a wider range of features and support, including customer service.
- Ease of Use: They are often more user-friendly and require less technical expertise.
- Data Security: Commercial solutions typically have better security features to protect sensitive patent data.
The choice between open-source and commercial solutions depends on the specific needs of the user or organization. Many businesses opt for commercial solutions, while individual researchers and smaller organizations may find open-source tools sufficient.
User Experiences and Reviews
User experiences and reviews play a crucial role in understanding the effectiveness of AI-powered patent analysis platforms. Before adopting a particular tool, it’s essential to assess its reputation and user satisfaction.
AI-driven patent analysis platforms often have user communities or forums where users share their experiences, best practices, and challenges. These resources can provide valuable insights and guidance for potential users.
Real-world feedback from users can shed light on various aspects, including the platform’s user-friendliness, the accuracy of its search results, the quality of AI-generated insights, and the level of customer support provided by the platform’s developers.
It’s worth noting that while reviews and user feedback are valuable, the suitability of a platform may vary depending on individual needs and the nature of the patent analysis project.
As we’ve explored the tools and platforms, it’s evident that AI has introduced an array of options for patent analysis, making the process more efficient and insightful.
Leave a Reply