AI is unlikely to completely replace venture capital firms but could change their nature of work.
Venture capitalists are typically responsible for identifying investment opportunities, conducting due diligence on potential investments and helping portfolio companies to grow and succeed. AI may automate certain tasks, but it will not replace human creativity and judgment necessary for venture investing.
AI can certainly streamline and improve certain aspects of venture capital. AI, for example, could be used by analysts to identify patterns and trends in large data sets that humans might miss. It could automate the tedious and time-consuming parts of due diligence checklist such as document reviews.
While AI is likely to have a significant impact on venture capital, it will not eliminate the need for expertise and judgment.
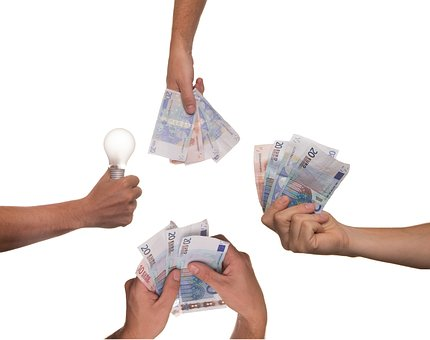
How AI will Reduce Need For Venture Capital Associates?
Artificial intelligence, machine learning and predictive analytics are revolutionizing workflows across industries, speeding up content creation and providing valuable insight from vast amounts of data.
Venture capitalists remain essential in the startup ecosystem despite these advances. They provide startups with financial resources, tools and connections to enable them to expand and succeed.
1. Artificial Intelligence
Artificial intelligence (AI) is the capability for machines to perform tasks that humans would normally complete manually. AI has revolutionized many industries, from transportation to customer service and beyond.
Bots are also helping organizations automate core business processes to save time and costs and enhance performance. For instance, bots can monitor customer portals or corporate email inboxes for new sales orders, automatically process them according to predefined business rules without human intervention required – all with the power of automation!
Another key area that could benefit from automation is shipping and handling. Vendors usually prepare shipments with bill of lading documents, carriers accept them and provide freight bills, the vendor then initiates a purchase order, delivers the shipment to its customer, and validates that receipt against the purchase order.
Automating these workflows with AI can reduce costs, boost efficiency and free employees to focus on higher-value activities. But businesses must approach this task strategically.
To maximize the potential of AI, companies must be able to quickly train algorithms on various types of data – not just structured but unstructured as well as social media content. This ensures that companies have access to a diverse selection of sources for training algorithms.
In addition to training AI on a large amount of data, it’s essential for teams to be able to quickly select and configure the right algorithm for any business problem or task at hand. This can be an extensive and costly process, so having automated algorithm design techniques in place helps streamline this step.
Companies uncertain of how to utilize AI should start with small projects such as content creation to test out and learn how their data can be utilized for it. After doing this, they can make an informed decision regarding which technology best meets their requirements and objectives.
Companies that understand both the potential advantages and drawbacks of AI can be sure they’re using it efficiently to drive growth, improve customer experience, boost staff productivity, and more. By implementing AI into their operations, businesses free talented employees from repetitive tasks that take up much of their workday, leaving them more time to tackle business problems and build customer relationships.
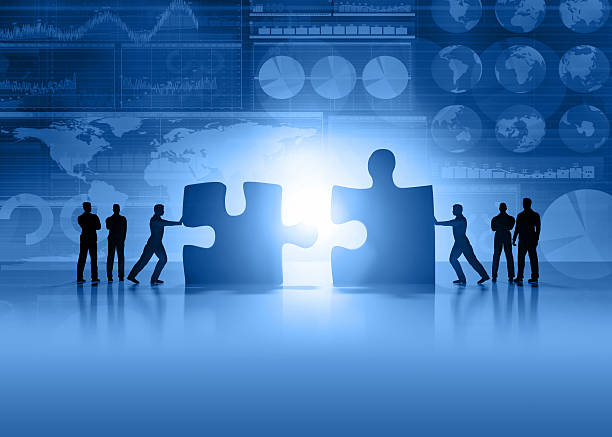
2. Machine Learning
Machine learning (ML) is an area of research that allows computers to learn without being explicitly programmed. This enables machines to extract actionable insight from vast amounts of data without human intervention.
Machine learning algorithms in factories, for instance, can monitor workflows across the facility and identify inefficiencies. They then self-correct to boost efficiency even further.
Machine learning (ML) algorithms can also predict outcomes based on a vast amount of data, such as stock prices or house values. In this way, ML helps companies make informed decisions by providing them with real-time insight into trends and opportunities.
Machine learning promises to revolutionize many industries, but it also presents businesses with a number of obstacles when trying to take advantage of its capabilities. These include bias in data, high costs and lack of expertise.
Businesses can still reap the rewards of Machine Learning (ML) if they’re willing to invest time and resources into deploying it. According to Algorithma’s survey, most companies spend between 8-90 days deploying a single model.
Furthermore, machine learning relies on data being continuously collected by models and fed back into them to keep them informed about their environment. This cycle, known as a feedback loop, is essential for making machine learning effective.
Machine learning in the future will assist companies in creating better content for customers, decreasing fraud and boosting security. Furthermore, organizations can optimize their business processes by monitoring risk areas and potential threats.
Businesses may need to adjust their approach to automation and data management. They should focus on building a foundation of data, developing machine learning algorithms (ML), applying them to real-world use cases, and setting best practices for deployment and upkeep of ML systems.
To realize the full potential of Machine Learning (ML), businesses must create and execute a strategy that extends far beyond pilot stage deployments. To do this, one must have an accurate understanding of goals and requirements as well as an actionable roadmap for building the necessary capabilities to meet those targets.
3. Predictive Analytics
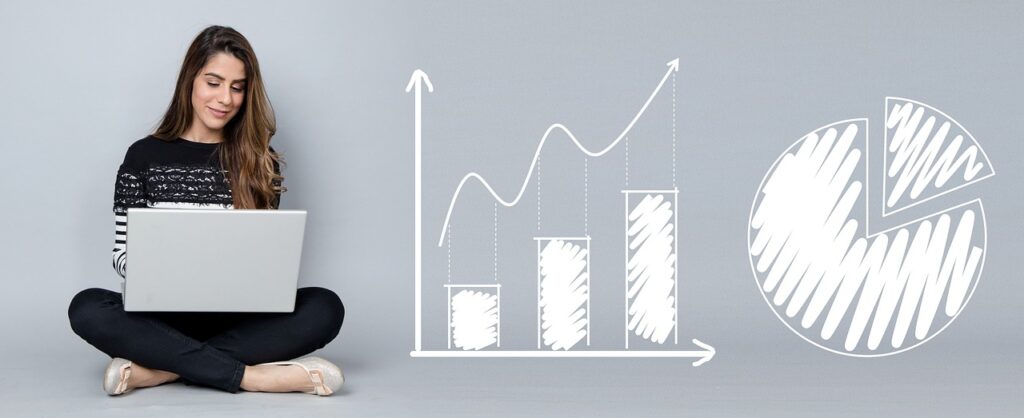
Predictive analytics is a broad field of data analysis that uses statistical techniques, machine learning techniques and other artificial intelligence technologies to uncover patterns in historical data, build predictive models and calculate the likelihood of future outcomes. Organizations and institutions can utilize predictive analytics to better understand risk, set clear benchmarks and make informed decisions about their business operations.
Predictive analytics is revolutionizing workflows across industries by speeding up content creation and extracting actionable intelligence from large, unstructured data sets.
AI-powered software
Businesses can benefit from AI-powered software by automating mundane, time-consuming tasks that require human input. Examples of such automated processes include creating customer email campaigns or cross-selling products to increase profitability.
Predictive analytics is becoming a widely adopted trend in business, and companies across many industries are using it to enhance their operations and gain competitive edge. From financial services and healthcare to cybersecurity, transportation or manufacturing – AI-powered tools are supporting an array of use cases which can reduce costs, boost efficiency and guarantee business success.
Businesses must first collect and organize the correct data to achieve their objective. This could include customer purchase information, demographic details, product return data, sales channel/pricing info and market segmentation. After collecting this information, businesses can then perform classification and regression – data science methodologies which assign categories to data sets and predict outcomes based on those categories.
Finally, they can construct their models using various algorithms such as linear and nonlinear regression, decision trees, neural networks and others. These programs use data to learn from experience so that they can make increasingly precise predictions in the future.
Retailers and marketers use predictive analytics to identify which customers are likely to purchase certain items based on their purchasing history. These insights enable them to better tailor their marketing efforts and offer more tailored products.
Predictive analytics is becoming an increasingly valuable tool for businesses, but there can be risks involved. One major one is overreliance on data which could lead to inaccurate models or overly optimistic outcomes.
4. Transforming Workflows
AI-driven workflows eliminate the need for human oversight, helping to guarantee processes run efficiently and consistently. Furthermore, these automations give key team members instantaneous access to task statuses as well as the ability to detect problems or bottlenecks before they become major issues.
Companies who incorporate AI-driven workflows into their systems typically experience significant time and resource savings. This is because AI-driven workflows automate tasks, send alerts, trigger processes – they also minimize errors and protect your company’s data from potential leaks or mishaps.
Machine learning algorithms are capable of learning and making decisions based on vast amounts of data, enabling them to improve over time. They even make predictions which humans would find difficult to anticipate, such as when and where a company might experience significant growth opportunities.
One example of how AI impacts venture capital practice is by aiding VCs in screening and identifying promising investments. To do this, VCs use a company’s data and other resources to develop an algorithm that determines if the venture has any chance for success.
This can be an incredibly powerful asset when combined with a compelling narrative for an investment. The most successful tech companies have an engaging storyline that unites technology and inspiring leadership to generate value for investors.
Recent research indicates that 75% of investment decisions in venture capital will be driven by AI and data analytics by 2025, signaling an important shift in how the industry functions and the role technology plays.
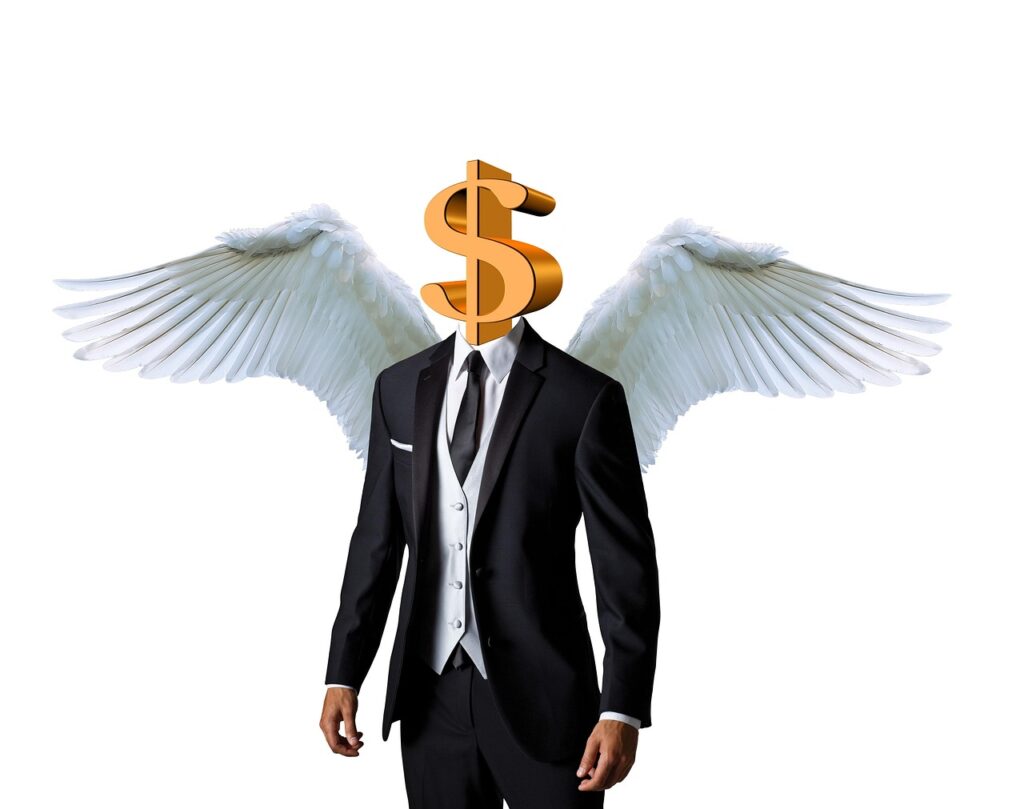
The Future of Venture Capital
Venture capitalists are witnessing a resurgence, with startups and investors alike eager to invest in innovative businesses. This trend can be attributed to several social developments such as the growth of global markets and an increasing focus on entrepreneurship.
One of the major trends in venture capital is the use of alternative data sources to make investment decisions. Previously, investors relied on traditional information like company financial statements and stock price movements; however, today they can access a vast wealth of information which allows them to identify distressed firms and create models that predict growth potential.
Another trend in venture capital is the increasing influence of institutional investors. Sovereign funds and other notable private equity firms have entered the space, seeking returns in a low-interest rate environment while participating in large-ticket deals.
One major reason is the rise of “unicorns.” These startups that are valued at more than one billion dollars and considered highly disruptive by many are sought out by investors who hope to acquire a significant portion of their value before they go public.
Funds such as these have brought more liquidity into the venture capital (VC) market and transformed how companies raise capital. Furthermore, they are emphasizing liquidity preference deals which enable VCs to receive a higher multiple when selling their shares in a company in the future.
Though these new investments have provided the venture capital industry with a boost, they also created some challenges. The market is saturated, making it difficult for smaller players to break into the fray.
There are a number of nuances that must be understood in order to be successful with venture capital funding. These include selecting the ideal time to raise, identifying investors who will provide value, and guaranteeing that businesses can recoup their invested funds.
With all the changes in venture capital, it is essential to remember that a successful investment requires hard work and dedication from entrepreneurs and their team. The VC’s role is to assist them in reaching their objectives while providing resources for growth.
AI Impact on Venture Investor Associates
AI has become a vital tool for venture capital firms, helping them identify and fund startups with the greatest potential. As such, there has been an uptick in investment into this space.
No doubt, technology is revolutionizing how we think, work and live. But how is this impacting venture capital practice?
One way VCs are utilizing AI for investment decisions is machine learning. This automated process utilizes artificial intelligence to expedite data analysis, thus shortening the time it takes to determine if a startup will be successful.
Another way VCs are utilizing AI is through predictive analytics. This process uses historical data to make predictions about future trends, as well as making general market forecasts.
The issue with this approach is its potential bias. Bias can manifest in various forms, such as gender or race.
Fortunately, there are ways to prevent this. Venture investors can utilize companies such as OpenAI for access to objective AI experts and capabilities.
For instance, the firm offers a machine learning model that assists seed-stage investors in making investment recommendations. It utilizes 20 factors like investors’ conversion rates and total money raised to identify the most promising seed investments.
However, if AI is used discriminatorily, it could have negative consequences for historically marginalized communities. For instance, it could reduce the amount of funding women-led and minority-led startups receive, leading to detrimental effects both on their businesses as well as on the general economy.
AI Impact on venture capitalists
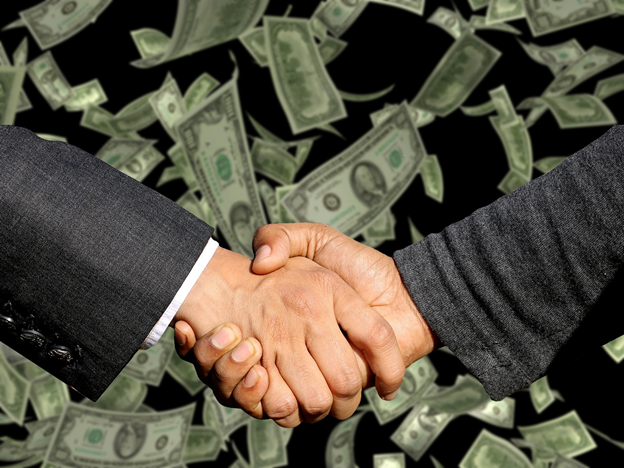
One of the key advances is that AI now allows principals to draw upon past data when making investment decisions. This is an especially useful feature since venture capitalists (VCs) often face numerous startup applications with various business models and growth strategies. Utilizing AI not only saves VCs time and energy in evaluating each application, but it also permits higher returns on capital than human analysis could offer.
Research reveals that venture capitalists who employ AI become better at recognizing startups similar to successful past successes, yet less likely to fund breakthrough businesses. This is likely because AI uses historical data which provides useful insight into companies with a history of success but not about startups developing innovative new businesses.
Another intriguing finding is that principals’ effects go beyond student achievement, impacting student attendance and suspension rates as well as teacher satisfaction and retention. It has been speculated that this might be because effective principals do more than what was previously thought – they engage in instructional leadership activities, create a productive school climate, encourage collaboration among staff members, manage personnel resources strategically – all without neglecting instructional leadership activities.
These findings indicate that investing in effective strategies to recruit and retain high-performing principals has significant rewards. They also underscore the necessity of diversifying the principal workforce and figuring out how best to retain top leaders.
Furthermore, the report emphasizes that VCs can mitigate their risk by taking steps to guarantee AI is only used as an aid to humans in decision-making and users have full transparency of what AI is doing with their data. This includes minimizing the amount of personal information AI collects and obtaining user consent before it is utilized.