In the dynamic landscape of today’s financial markets, the regulation and enforcement of securities laws have become increasingly complex and challenging. Securities laws, designed to safeguard investors and maintain market integrity, are subject to constant evolution, driven by global economic trends, technological advancements, and evolving market dynamics. To meet these ever-evolving challenges, the legal and financial industries are turning to artificial intelligence (AI) as a powerful tool to navigate the intricate web of securities regulations, detect fraudulent activities, and ensure compliance.
AI, with its capacity for rapid data processing, pattern recognition, and predictive analytics, is poised to revolutionize the way securities laws are analyzed, interpreted, and enforced. This transformative technology holds the promise of enhancing regulatory compliance, improving risk management, and strengthening the mechanisms for monitoring and investigating securities-related misconduct.
Machine learning uses large data sets to discover associations. These associations can then be used to predict future events. Machine learning can be used to reduce costs, improve accuracy and speed up processes when analyzing securities regulations.
There are still some challenges to overcome. It can be used to improve securities regulation analysis through automation of tasks and enhanced compliance monitoring.
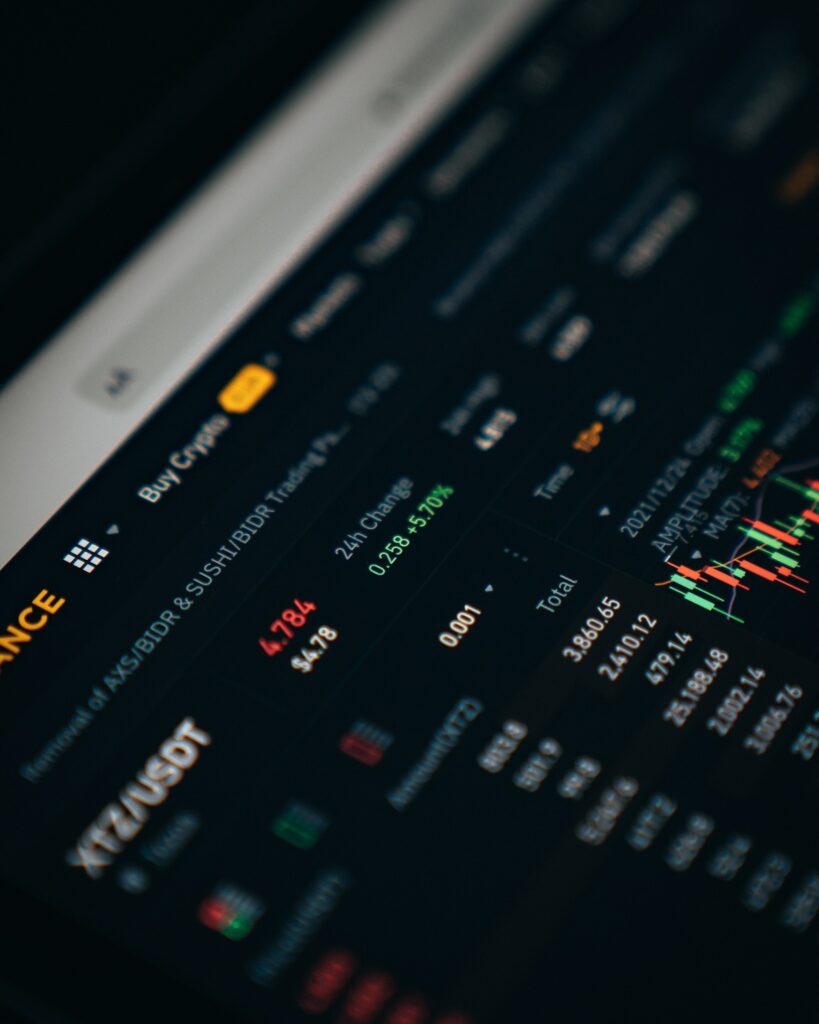
1. Risk Assessment
Model risk management is used to identify risks that may be associated with trading behaviors, such as excessive trading or unprofitable transactions. Model risk management is the use of machine-learning for this purpose. It can be achieved by stress testing existing market models, or identifying emerging threats in new model outputs. Avoiding overfitting and drifting models is important. These can happen when a model learns idiosyncratic patterns from its training data, and fails to generalize them well to new data.
Machine learning, which uses advanced computational technology, has enabled securities regulators and other stakeholders to detect fraud more quickly and efficiently. A machine learning algorithm, for example, can analyze past investigations in order to identify possible indicators of securities fraud. The algorithm can be used to analyze new information such as SEC filings to predict fraud. This can ensure that market regulation is enforced effectively.
Machine learning can also be used to analyze the risk rankings of assets in order to identify those that are vulnerable. This information can be used to apply risk-based security control to the system in order to mitigate these risks. Machine learning can also be used to assess the impact of vulnerabilities and threats on the criticality a business operation. It can be used to monitor the changes in the threat environment and determine whether any mitigating control measures need to be added or updated.
Although this technology is rapidly advancing, it has not yet been fully implemented by many organizations. This is due to a number of reasons. The sheer volume of data to be processed is one reason. It would be impossible, using traditional methods to find relevant patterns in all this data. The ML algorithms are able to process large data sets quickly and accurately. It is much easier to prioritize and find the most relevant information. A second reason is the limited ability of traditional methods to identify latent factors, such as fraud probability. ML can better recognize latent variables that are difficult to detect by humans.
2. Trade Settlement
The settlement of trades is a vital part of the stock exchange. The entire trading system would collapse without it. By reducing the amount of time required to flag anomalies for review and identify them, machine learning can improve the efficiency in the settlement cycle. The result will be fewer restrictions and violations for traders.
Machine Learning is also able to detect patterns that indicate fraud or misconduct. The algorithms detect disclosures that are unusual or indicate a risk of misconduct. Researchers can also use case files to create an algorithm which can predict the probability of investment fraud within a particular industry or market. This analysis will help regulators, Dealer members and other stakeholders focus their investigations in high-risk areas.
Machine learning is a popular tool among financial firms because it helps them create efficiency, reduce costs and improve performance. There are challenges in implementing the technology. This is particularly true in the financial industry, which generates large amounts of data. Machine learning can help sort and sift these large datasets quickly.
This technology is best demonstrated by robo-advisors. These online services provide users with a customized portfolio of investments based on their risk preferences. These services are available since a long time, but they continue to improve and become more sophisticated.
JPMorgan has filed a patent with U.S. Patent and Trademark Office to create a new AI powered service called IndexGPT. This platform will use the Generative Pre-Trained Transformer to analyze user inputs and suggest investment strategy based on risk preferences.
These technologies are rapidly advancing, but there is concern that they may introduce bias in the data. It can be a problem for businesses that use them. If an algorithm was designed to put the interests of one firm or advisor above those of its clients, this could be a violation of fiduciary standard. Also, people who label data for a machine-learning model could be biased, and this bias may reflect in the generated model.
3. Asset Management
Asset management is evolving, and so are the tools that manage assets. Data collection, analysis and processing are done using machine learning algorithms. This allows financial firms to make accurate predictions and identify new investment opportunities. These models can provide early warnings of potential risks. They are therefore a valuable tool to regulators who want to protect investors.
Machine learning is being used to ensure regulatory compliance. SEC staff, for example, uses a variety machine learning methods to identify suspicious activity that could warrant a further investigation by staff in enforcement or examination. Automated analysis is also being used by staff to prioritize examinations of financial advisers. This involves identifying regulatory filings which indicate a higher risk of misconduct and violations of SEC regulations. These algorithms are better at identifying files that need further review than random, but can also produce false positives, or as we call them more colloquially, “false alerts”. Due to this potential for false alerts, staff examiners are continuing to critically scrutinize the output of these models.
Machine learning applications of different types are being developed in order to improve other regulatory processes. Automated analysis, for example, is used to detect securities fraud and insider trades by identifying patterns of behavior in market participants. The results are compared to historical patterns in order to detect anomalies. Staff can then be alerted when the pattern changes and suggests possible illegal activity.
Machine learning skills are in high demand as the use of artificial intelligent in finance continues to grow. There are many ways to become a data analyst within the financial services industry. You can become a data analyst by completing a degree, pursuing specialized qualifications, or gaining relevant experience. Many businesses are also struggling to find qualified workers, and so they must offer higher wages in order to attract them.
4. Reporting on regulatory matters
Machine learning (ML) can be used to improve regulatory reporting accuracy and efficiency. Here’s an example of how ML can streamline regulatory reporting:
Data extraction
ML is a powerful tool for extracting data from various sources such as financial reports and transaction records. Natural Language Processing (NLP), a technique that parses unstructured data, can be used for accurate reporting.
The ML algorithm will automatically validate and clean the data by checking for errors, inconsistencies, and missing information. Data in regulatory reports are accurate and reliable.
ML can convert data to the format needed for regulatory reporting, which includes templates and structures. It can be done automatically, saving time and reducing the chance of human error.
Monitoring of Regulatory Rules
Models can continuously monitor changes in regulatory rules and requirements. ML can help identify the impact on reporting obligations when regulations change. This helps ensure that all reports are compliant.
ML can implement quality checks for data and reports. It can flag up any anomalies or inconsistent outcomes for human review. It is crucial to maintain data accuracy and regulatory compliance.
The ML tool can be used to schedule and trigger reports based upon predefined criteria. This will reduce the chance of missing deadlines and ensure timely submissions.
Prediction and correction of errors
Machine Learning models can use historical data to predict potential reporting errors. The error can be corrected before submission. The ML algorithm analyzes historical data in order to optimize the reporting processes. This may involve identifying bottlenecks, reducing the manual effort and improving efficiency. ML can identify problems in real time and send alerts when deviations occur.
ML can create and maintain a audit trail of all reporting activities. It is easier to trace and verify the reports. ML automates regulatory reporting to reduce the chance of errors and frees human resources for more strategic and analytic tasks. It is also a great way to adapt quickly to new regulations, since ML models can be easily updated.
Remember that while ML can be a powerful tool for regulatory reporting and compliance, it is often necessary to combine technology with human oversight. The human must be involved in the process to provide context and interpret results. They must also adhere to ethical and regulatory standards, and ensure that ethics is adhered.
5. Fraud Prediction
Financial institutions face a constant challenge in detecting fraud. The traditional methods of fraud detection are based on rules-based systems, which flag suspicious transactions according to predefined criteria. They can be rigid and need to be updated frequently, since fraudsters are constantly developing new schemes. Machine learning analyzes data in order to detect patterns and anomalies. These algorithms are able to predict fraud much more accurately than previous logic-based methods.
It is particularly important for a highly regulated industry like the securities market. Machine learning can help reduce the time it takes to detect and investigate fraud, which allows regulatory authorities to act quickly to protect investors and maintain market integrity.
Recent research has applied machine learning in order to detect investment fraud in the Canadian securities sector. The study used cases heard by Investment Industry Regulatory Organization of Canada between 2008 and 2019 Researchers trained the model to predict if an individual’s behaviour was likely to be fraudulent. The researchers tested the model’s performance using a series of test cases. They found that it was five times as accurate as random in identifying language within investment adviser regulatory filings which could warrant referral to enforcement.
The study found that certain features are critical in predicting fraud. The variables that were most significant included the offender’s experience, whether the investor was retired, the amount invested and lost, and the net worth of the investors. Investigators searching for fraud can use the top five predictors as a guide. The performance of the model was dependent on the amount of data used for training. The model can identify more associations with larger datasets, which results in a higher accuracy of prediction. A model that is “overfitted”, and therefore overestimates the likelihood of fraud, can be caused by smaller training sets.
To avoid this, researchers developed a cost-sensitive supervised model that reduces the likelihood of incorrectly classifying an observation as fraud. The IIROC data was used to test the supervised model, which achieved a recall score of 97%. The random forest models with GridSearch and the base random forest were the most accurate classifiers. They demonstrated that they could identify all the fraud observations within the validation data without overfitting and underfitting. This is a significant finding because any misclassification could be costly to financial institutions.