Many insurers use AI, and customers reap the benefits of comparative shopping, rapid claims processing, and around-the-clock service. However, the technology can present challenges to regulatory compliance and business ethics. One major challenge is proxy discrimination and models that employ correlations that may reflect past discrimination. Strong governance and transparent model design help mitigate this risk. Here is how AI and Machine Learning helps in Insurance Law:
1. Automation of Claims Process
AI-powered tools are already reshaping the insurance industry. With the potential to reduce costs, improve efficiency, and enhance customer experience, insurers that embrace disruptive technology will be more competitive. This is especially true for companies that focus on creating opportunities from AI instead of viewing it as a threat to their business.
One area where AI is making an impact is in the process of settling claims. This involves reviewing the claimant’s policy, assessing the damage, and verifying information with third-party databases. Insurers can use automation tools to process claims faster and more accurately, reducing the risk of fraud while improving overall customer satisfaction.
Similarly, underwriting is becoming more efficient and accurate with the help of automated algorithms. These tools can quickly scan thousands of submissions, including ACORD forms, spreadsheets, loss runs, brokers’ emails, and more to identify red flags and determine whether a submission should be approved or rejected. This will save underwriters time and enable them to better prioritize the best submissions based on their risk appetite and underwriting triage guidelines.
The effectiveness of most AI technologies is dependent on the volume and quality of data that they can analyze. In order to realize the full benefits of AI in the insurance industry, carriers must invest in building a robust data strategy and architecture. This includes acquiring, organizing, and curating data so that it can be used for rapid analytics development.
The regulatory environment around the use of AI is evolving at a rapid pace. However, many regulatory bodies are taking a cautious approach to addressing the significant risks and opportunities associated with this exciting new technology. A proportionate approach to regulation is critical for enabling the safe and responsible adoption of AI by the insurance industry.
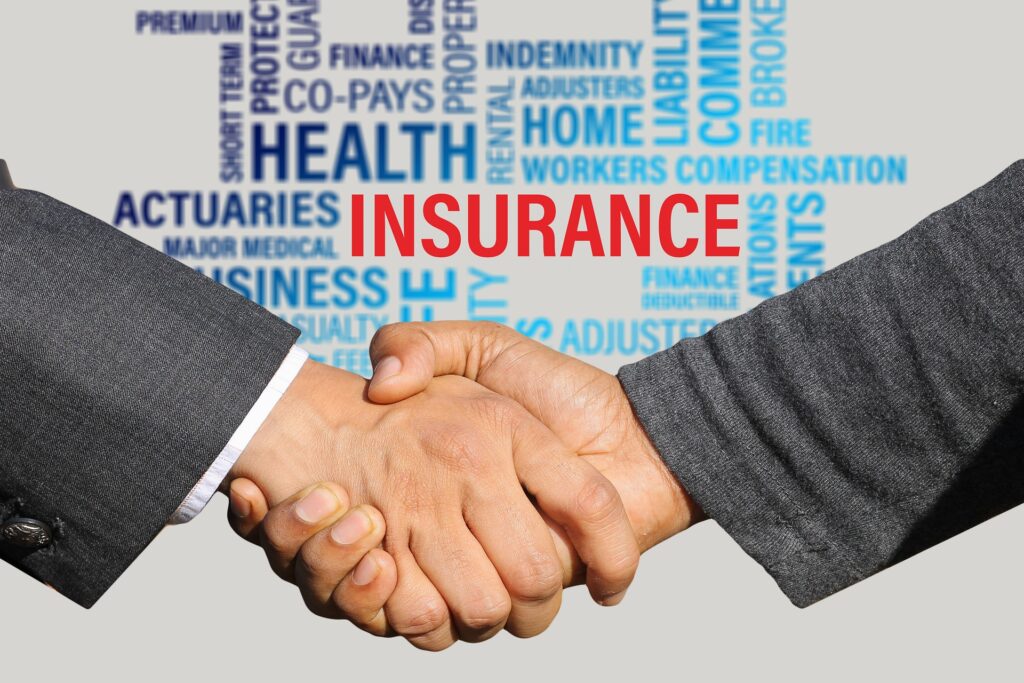
2. Predictive Analytics
The use of predictive analytics in insurance helps companies save time and money by enabling the industry to make better decisions. The technology identifies patterns and underlying trends in large data sets to predict future outcomes. Insurance companies are using predictive analytics in a variety of ways, including to improve the quality of customer service, reduce operating costs, identify fraud, and streamline claims processes.
Predictive analytics is a powerful tool that helps insurers anticipate the needs of customers and develop solutions that are customized for each individual. The technology makes it possible to assess a client’s risk profile without the need for human intervention by analyzing unstructured data, such as emails, social media posts, GPS location, video, images, and data from smart devices like telematics. The data analyzed is then used to create a model that will determine the likelihood of a claim or loss based on a number of different factors.
Insurance companies are also leveraging predictive analytics in the field of underwriting. For example, when assessing car insurance applications, behavioral data is assessed to evaluate risk. This includes analyzing how a driver slams on the brakes or how often they peel around corners. In addition, external factors are incorporated into the evaluation to determine the odds of a car being stolen, for example. This information is then used to set premium rates for vehicle insurance policies.
Predictive analytics helps to ensure that customers are happy with their policy by tracking their behavior and identifying potential churn. This is achieved by identifying and addressing issues before they become major problems. A predictive analytics tool such as Genesis Engage can track unsatisfied clients and help them find a solution before they leave for good.
3. Recommendation Engine
There are countless opportunities for AI to streamline and transform insurance processes. Insurance is a complex industry, and AI has the potential to automate many of its processes while delivering better, faster outcomes for both insurers and consumers. Using ML and automation technologies, insurers can optimize every function in their value chains from policy management to customer experience.
For example, a UK motor insurance carrier that uses DataRobot for pricing machine learning was able to reduce its losses, improve its combined ratio, and increase its retention rates by switching to a gradient boosting model. The change allowed it to deliver precise, risk-specific pricing and to mitigate its vulnerability to adverse selection.
The influx of data is changing the way insurers approach underwriting. Pogreb expects that the number of applications a human underwriter has to review will drop as AI technology is adopted. Instead of manually reviewing each submission, underwriters will be able to manage portfolios, focusing their attention on the most important cases.
AI is also helping underwriters make more informed decisions regarding the type of coverage an applicant should get. By analyzing a person’s unique circumstances and habits, AI systems are able to provide more accurate advice and recommendations. This will ultimately lead to more tailored and customized insurance products.
With the increasing demand for a hassle-free navigation experience, ML and automation technologies are rapidly transforming the insurance industry. Among the most promising developments is an AI-powered chatbot that helps customers resolve their queries. It combines machine learning, natural language processing, and knowledge bases to deliver a quality customer service while boosting insurers’ revenue and enhancing their brand image.
4. Customer Relationship Management
The insurance industry is sitting on a treasure trove of data that can be leveraged to increase customer engagement, provide better service and tailor products to meet specific needs. This can be done through AI, which is a category of advanced analytics that can help to improve customer experiences, drive operational efficiency and deliver business value.
AI can help to automate processes, reduce costs, and increase speed and accuracy, enabling insurers to move from a “detect and repair” framework to a “predict and prevent” model that will benefit customers. It is also allowing them to offer more personalized services and to reach new customers by offering new business models such as robo-advising.
However, the adoption of AI in insurance is not without its challenges. The industry is being disrupted by agile, fast-moving insurtech startups and forward-thinking insurance companies that are providing new AI-enabled services. Insurers have a long road ahead of them to transform their business models and become fully integrated with emerging technology.
Insurance companies should start focusing on the strategic use of AI in their businesses, taking a risk-based approach that prioritizes areas with lower regulatory risks and opportunities for greater productivity gains. They should also consider the need for adequate controls and governance structures, including a single overarching manager with responsibility for all AI systems across the company (e.g., an appointed “AI officer”).
Insurers should also be considering how they will manage and monitor the ethical, privacy, and cybersecurity implications of their AI systems. For example, if an AI application picks up on gender or racial bias from historical underwriting decisions, it could have negative consequences. This is why the National Association of Insurance Commissioners is working on a Model Insurance Regulation Bulletin that will guide companies in setting up their governance systems and regulatory expectations for AI products.
5. Litigation and Dispute Resolution
AI has made great strides in helping with document management, dispute resolution and predictive analytics, including in insurance-related cases. Take a look at the ways in which AI can improve these legal processes.
Document Management and Discovery
- Efficient data processing: In insurance litigation parties are often required to sort through large amounts of information, such as contracts, policies and emails. Medical records, too, can be included. AI-powered software is able to quickly categorize and index these documents in order to make them searchable.
- Natural Language Processing: AI algorithms, in particular NLP algorithms can analyze and understand documents by extracting important information, identifying clauses or words, and summarizing long texts. This speeds up the document review process.
- Reducing human error: Automating document review tasks can reduce the risk of human errors and oversights in identifying evidence or legal issues.
- Cost savings: AI driven document management and discovery could lead to significant cost savings as lawyers spend less time manually reviewing documentation.
- Predictive Code: AI predicts which documents will be relevant in a particular case. This helps to prioritize review efforts, and allocate resources more efficiently.
Predictive analytics for settlement negotiations
Data Analyses
AI can analyze case data from the past, court decisions, and other information relevant to identify patterns and trends. This can give valuable insight into similar cases that have been solved in the past.
Risk Analysis
AI powered predictive analytics can help legal professionals and insurers evaluate the risks of proceeding to trial or settling.
Optimizing settlement offers
Legal professionals can make informed decisions regarding settlement offers when they have predictive insights. AI can be used to predict the likely outcome of a trial and guide negotiation in order to reach a more favorable settlement.
Time efficiency
Predictive Analytics can speed up settlement negotiations, by giving parties a realistic assessment early on in the process. This reduces the need for lengthy legal battles.
The use of AI to predict likely outcomes can add transparency to negotiations since both parties are able to rely on data-driven predictions instead of purely antagonistic positions.
It’s still important to keep in mind some limitations and considerations:
- Data Quantity: The accuracy and quality of predictive analytics is dependent on the quantity and quality of historical data. Data may be limited in some cases and lead to less accurate predictions.
- Legal and Ethical Implications While predictive analytics is a useful tool, it can raise ethical and legal issues when AI is used to make decisions, especially in situations where human judgment and expertise are required.
- Fairness and Bias: AI can inherit biases based on historical data. This could lead to unfair outcomes. Predictive analytics should be used to ensure fairness and mitigate bias.
AI can significantly improve the efficiency and effectiveness in litigation and dispute resolution for insurance cases. It can speed up discovery and streamline document management. It also provides valuable insight for settlement negotiations. It should, however, be used with caution, with an understanding of its capabilities, as well as limitations. This is always done in conjunction with legal professionals. AI should be used in a way that is ethical and fair.
6. Risk Assessment
AI is increasingly adept at performing tasks that were historically difficult for computers to execute like recognizing images, identifying spoken words and parsing unstructured data. This is driven by higher computing power, memory capacity and cloud computing technologies, combined with improved algorithms, better programming language support and global connectivity.
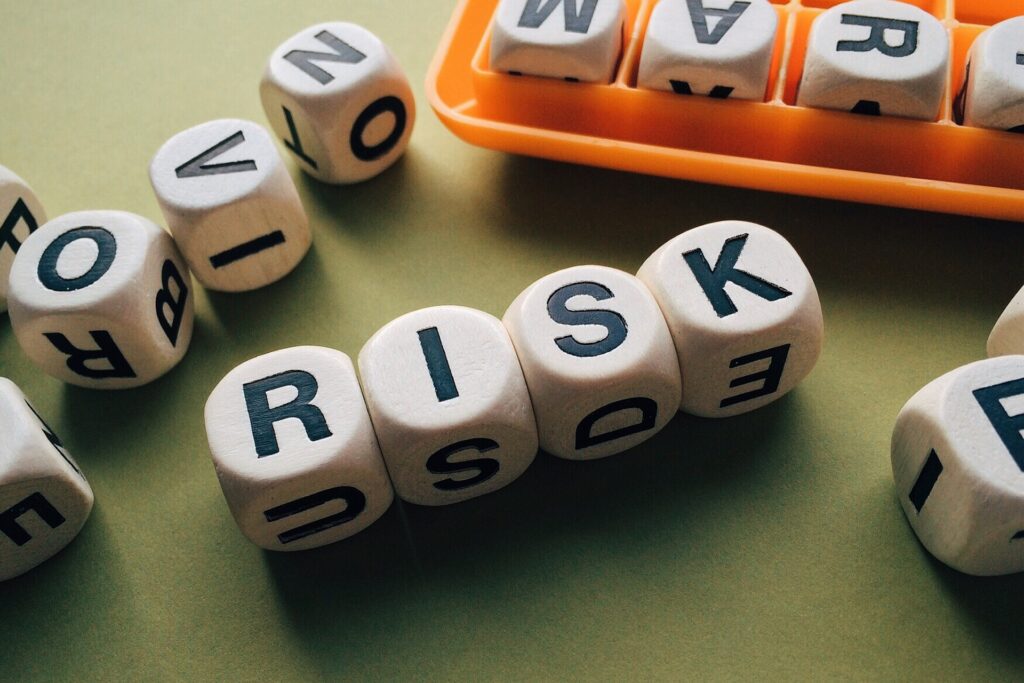
The insurance industry is already using AI to assess risks, including underwriting and claims processing. For underwriting, AI can streamline processes such as reviewing documents and determining eligibility by analyzing images, sensors, and historical data to determine what is covered under a policy. It can also help reduce the number of applications a human underwriter is required to handle.
In addition, AI can be used to assess insurance risks by analyzing large amounts of historical data to identify suspicious patterns that are not consistent with previous legitimate claims or customer behavior. This helps insurers more quickly investigate and resolve questions about illegitimate claims. It can also assist with detecting and flagging potential fraud, which is a significant risk to the insurance industry.
Despite the benefits of AI, it is important for insurers to understand its limitations and be mindful of potential regulatory issues. These include issues arising from the use of AI in retail business contexts (which gives rise to a potential moral hazard and micro-risk segmentation issue) as well as other issues arising from the use of ML to analyze personal data for underwriting purposes.
Currently, the National Association of Insurance Commissioners (NAIC) is working with industry leaders to develop model insurance regulatory principles on artificial intelligence. These principles will provide guidance to insurers in establishing governance systems and understanding regulatory expectations for the use of AI.